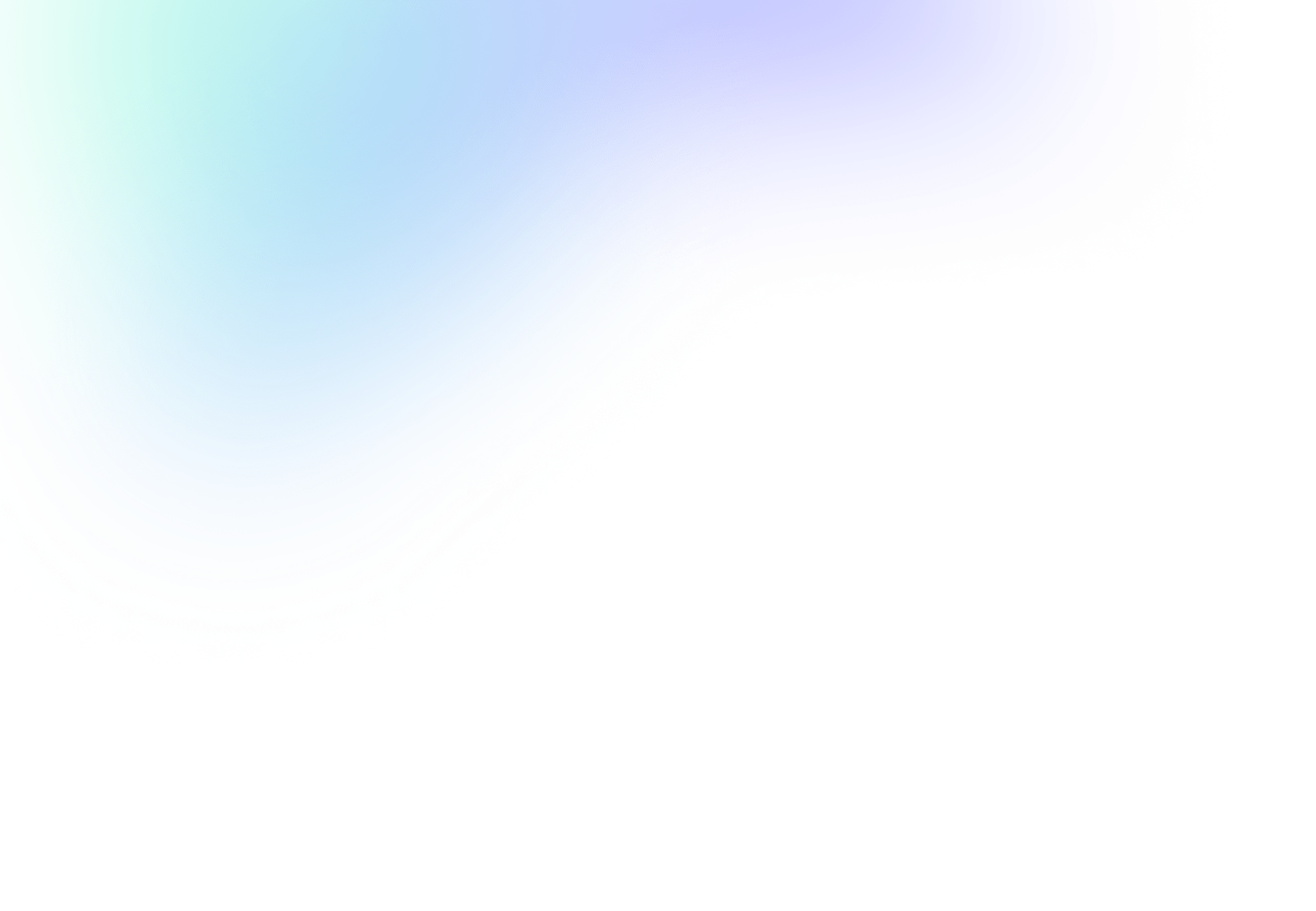
Deep learning was enabled by hardware, and its progress is now limited by hardware
Models and data set growth are increasingly outpacing hardware progress, making the job of the AI developer more complicated than a multidimensional game of Tetris. Moreover, when an AI developer is able to find that ephemeral best fit, the cost and power consumption are just plain unsustainable.
We have a visceral understanding of this problem because we have walked miles in the shoes of the AI developer. We know that the problem is multidimensional. We know that one or two improvements will not be enough. We have to reimagine computing from the lens of the AI developer. That means a true hardware and software co-design of a dynamic system that creates a step function improvement in performance, efficiency and developer productivity.
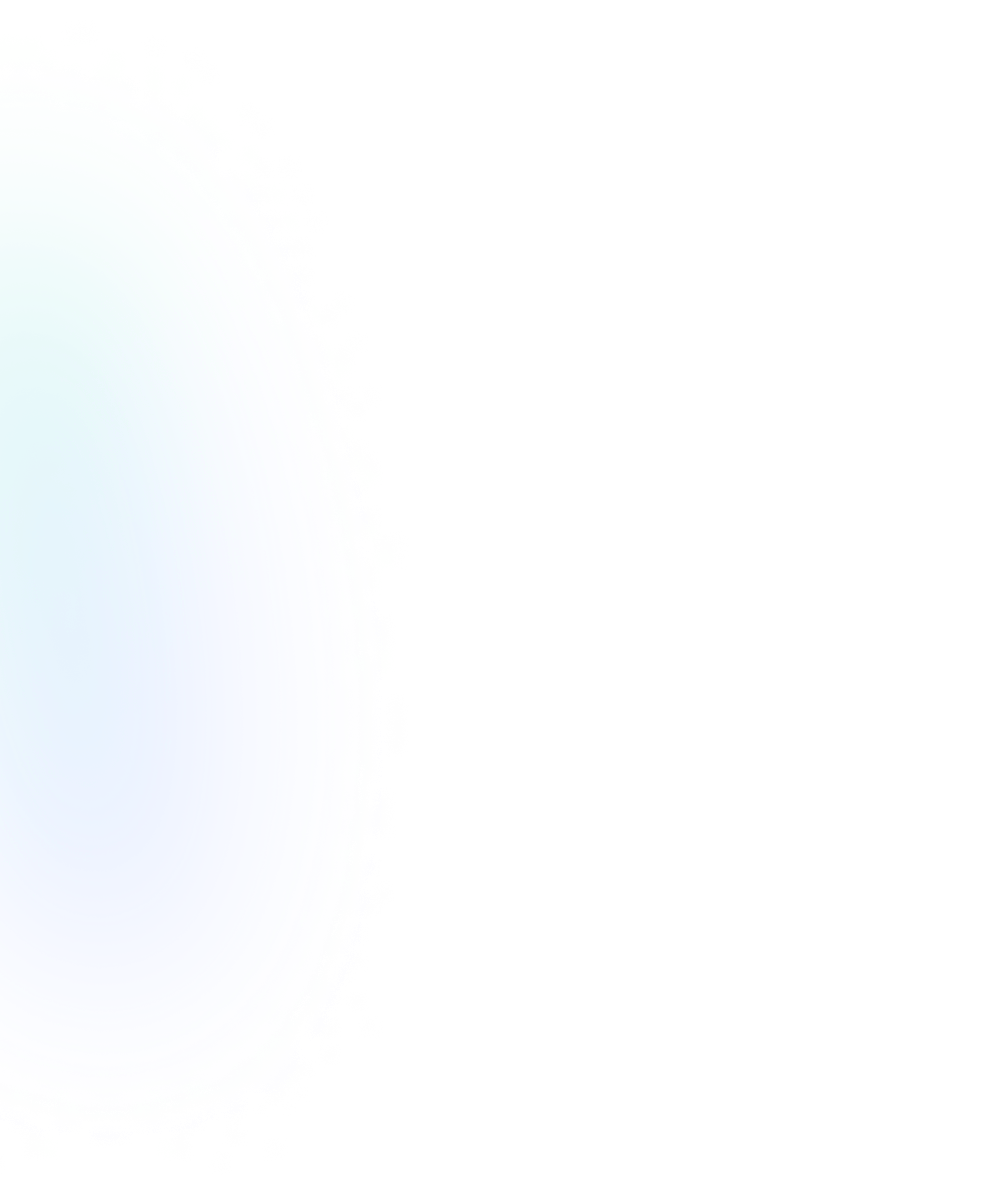
Our Innovations Stem from Thoughtful Hardware-Software Co-design
01
Dynamic AI Compiler
We meticulously studied the AI workloads and the current stack to understand the problems, and then looked at what the workload was looking for from hardware. We then designed a compiler around which we designed our computer architecture. Our compiler dynamically places tasks to maximize hardware utilization, making writing code for a 1000 node cluster as easy as for one. We give you a massive performance gain straight out of the box so that you don’t have to spend your valuable time doing low level optimizations. Whether you're training models or deploying a complex AI tool, our approach empowers you to achieve peak performance and unleash your true potential.

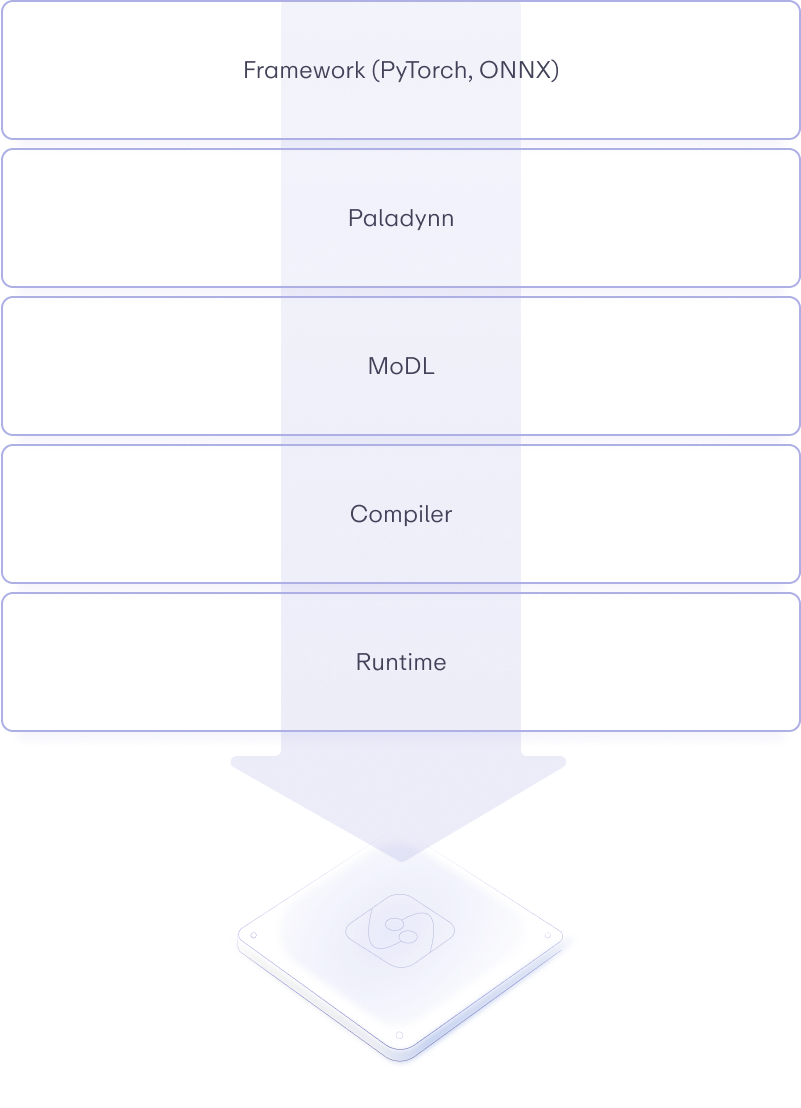
Co-designed system for a 20x increase in AI performance.
02
New Data Type for More Efficient and Reliable AI
We solved a 250 year old math problem to create a breakthrough in computing efficiency. Our innovative logarithmic data type not only has better representation than floating point, it enables an astounding 10x increase in efficiency, allowing us to break free from legacy approaches to parallel computing. By revolutionizing the way you compute, we empower you to achieve more with less.
.png)
8-bit number line spectra for FP, PAL, INT, LNS.
03
Near-memory Distributed Dataflow Architecture
The key to efficiency lies in better memory management. We took the conventional dataflow architecture and we took it a step further. Our tiered memory architecture optimizes data flow to maximize throughput and efficiency without sacrificing generality. Experience a whole new level of performance and efficiency that opens with intentional co-design.
.png)
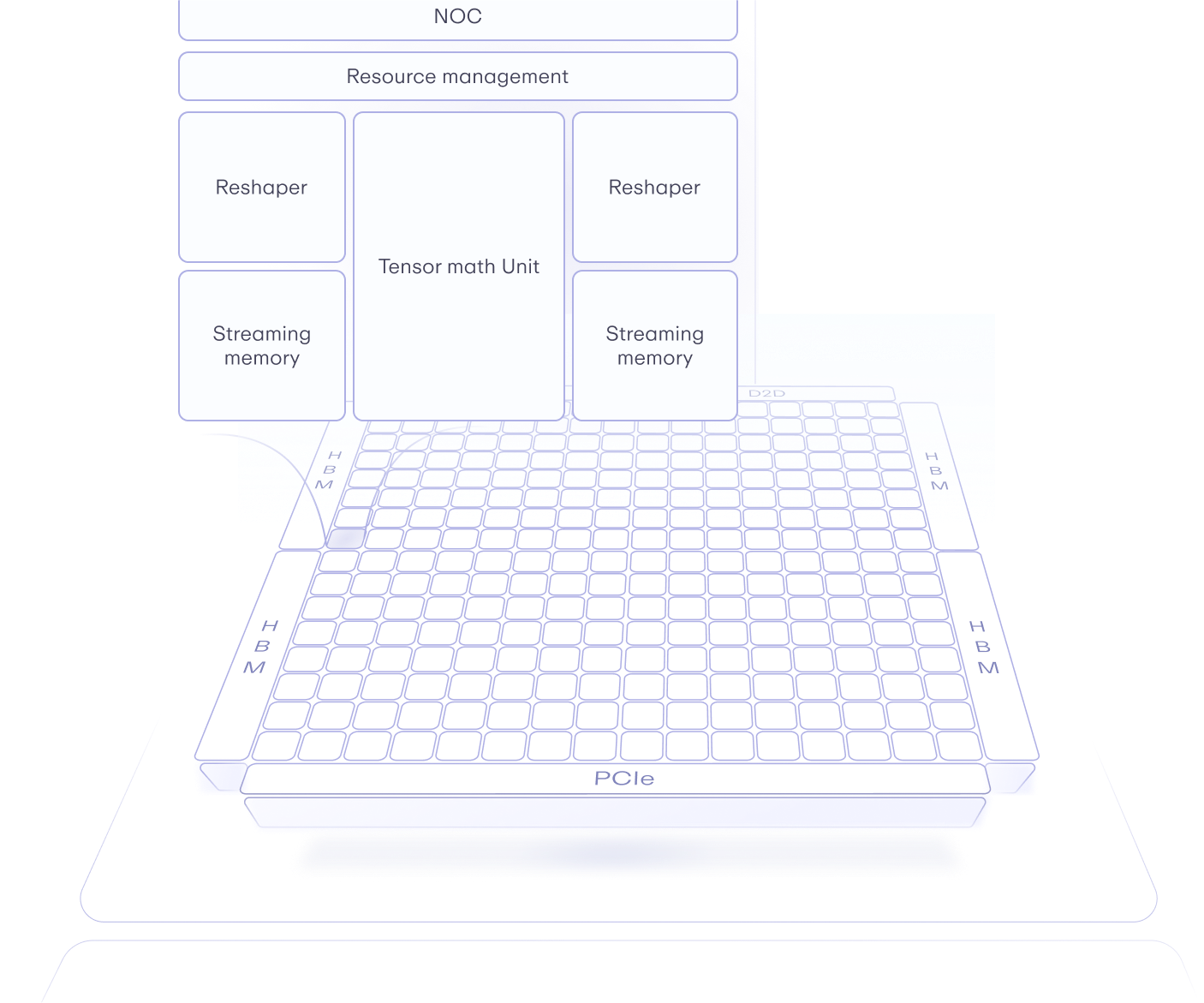
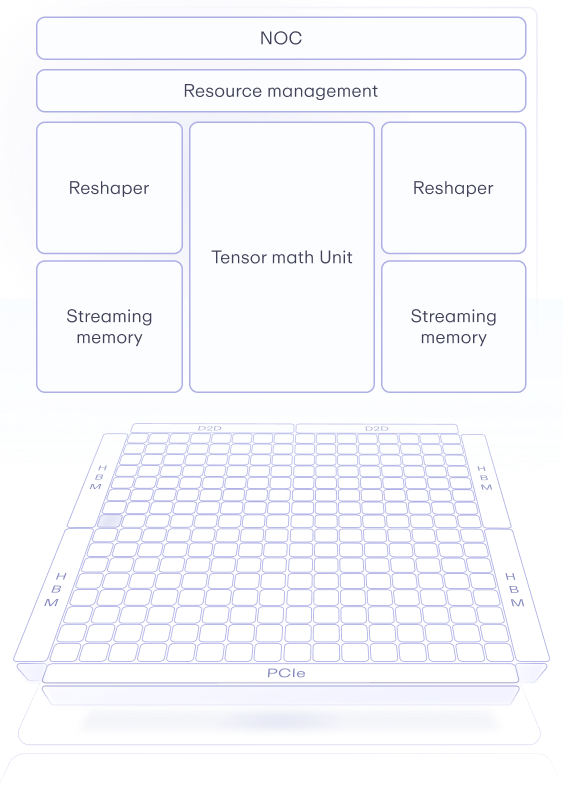
Scalable tile based architecture designed to meet the needs of AI engineers.